Speakers
‹ Back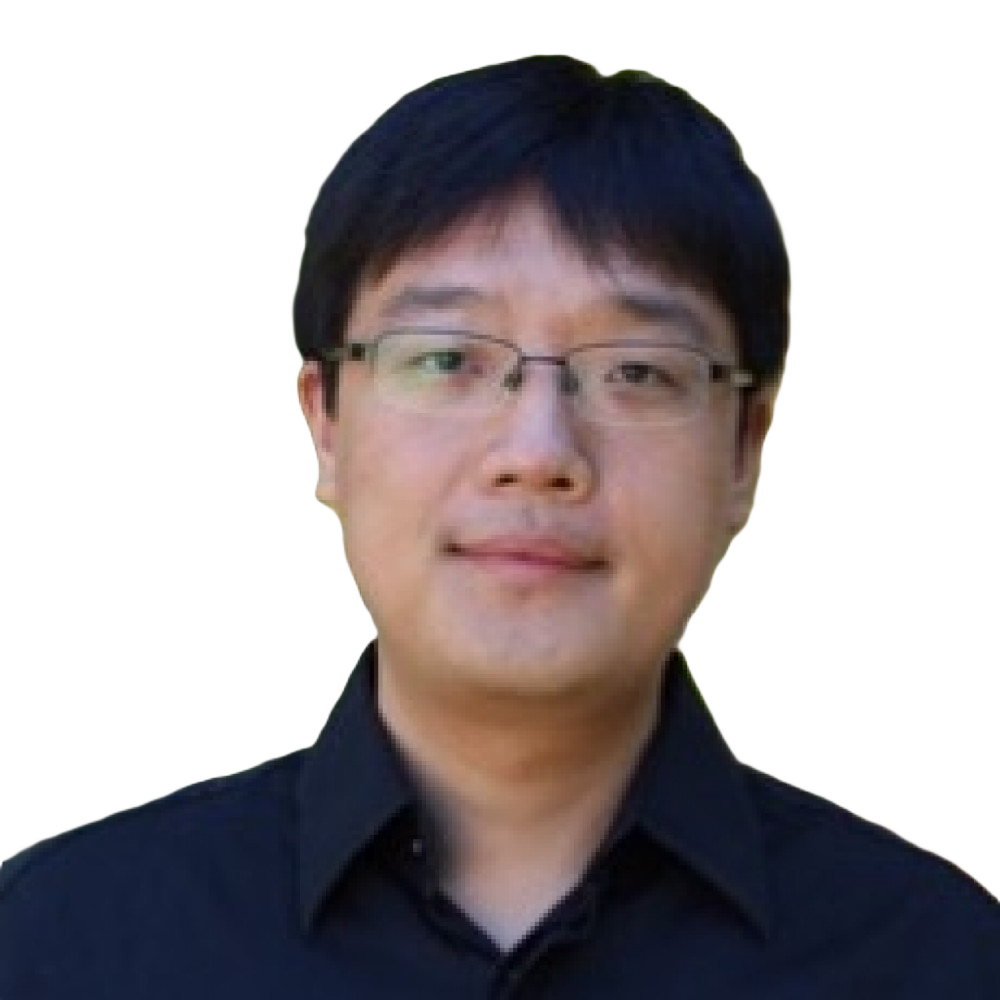
Prof. Yanan Sui
Associate Professor, Tsinghua University
CV
Yanan Sui (YananSui.com), Associate Professor at Tsinghua University, is dedicated to the research of human neuro-musculo-skeletal modeling and control, with applications in embodied intelligence and brain-machine interaction. He received his B.S. from Tsinghua University, his Ph.D. from Caltech, and did postdoctoral work at Caltech and Stanford University. His work on safe optimization has been included in textbooks at Stanford and other universities. He co-won the Best Conference Paper Award and the Best Paper Award on Human-Robot Interaction at the 2020 International Conference on Robotics and Automation. His work has been successfully applied to the clinical treatment of neural injuries in China and the United States. For his contribution to the interdisciplinary field of artificial intelligence and neural engineering, he was selected as one of MIT Technology Review's Innovators Under 35 in China.
Abstract
Abstract:Modeling and control of the human neuro-musculo-skeletal system is important for understanding human movement, developing embodied intelligence, and optimizing human-robot interaction. However, current models are restricted to a limited range of body parts and often with a reduced number of muscles. There is also a lack of algorithms capable of controlling over 600 muscles to generate reasonable human movements. To fill this gap, we build a musculoskeletal model with all of the body segments, joints, and muscle-tendon units, allowing simulation of whole-body dynamics and interaction with various devices. We develop a new algorithm using low-dimensional representation and hierarchical deep reinforcement learning to achieve state-of-the-art whole-body control. We validate the effectiveness of our model and algorithm in simulations using real human locomotion data. This work promotes a deeper understanding of human motion control and better design of interactive robots.